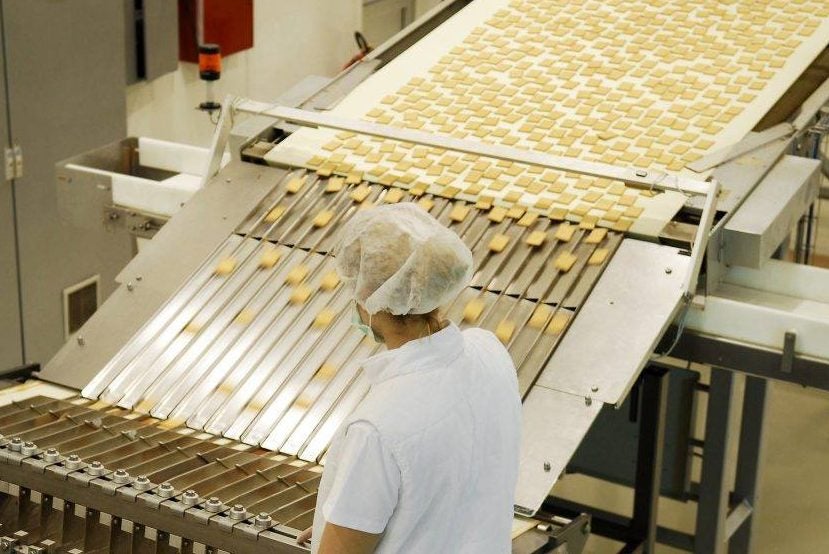
Liran Akavia, founder and chief operating officer of Israel-based tech firm Seebo, offers this simple piece of advice to food companies on how AI can make their plants more efficient and reduce food waste. “Food manufacturers,” he says, “should not limit their horizons to what is humanly possible.”
This counter-intuitive maxim may well raise a smile but will also pique the interest of companies ever seeking a competitive edge. And it perfectly encapsulates what AI, machine learning, and advanced data analytics offer food manufacturers in relation to waste reduction.
How well do you really know your competitors?
Access the most comprehensive Company Profiles on the market, powered by GlobalData. Save hours of research. Gain competitive edge.
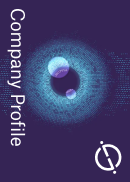
Thank you!
Your download email will arrive shortly
Not ready to buy yet? Download a free sample
We are confident about the unique quality of our Company Profiles. However, we want you to make the most beneficial decision for your business, so we offer a free sample that you can download by submitting the below form
By GlobalData“AI opens a whole new potential in the area of process efficiency,” Akavia insists.
Food companies have justifiably been captivated by the advantage that complex data analytics can provide in consumer marketing and AI is increasingly featuring in efforts to reduce waste at various points in the value chain, notably in retail and foodservice, and in the home.
How industrial AI can increase processing efficiency and reduce food waste has been less discussed, but its increasing application by major food groups speaks to its potential to precipitate a new wave of efficiency gains in the food industry and make a significant contribution to the global food waste challenge.
The fact that AI and machine learning are providing something new and beneficial to companies that have always made maximising processing efficiency a priority, is in itself highly significant. It is in these companies’ DNA to seek out inefficiency and its causes, but that vigilance had human limitations.
Major attraction
Working principally with manufacturers of baked goods, Seebo’s order book reads like a roll call of major multinational food groups, with companies such as Nestlé, Mondelez International, and General Mills prominently advertised as customers.
These companies are already “working flat-out to be as efficient as possible, particularly in preventing waste while maintaining high quality standards”, Akavia says. “The common challenge they all have is that every single one of them eventually hits a glass ceiling, and their KPIs start to elude them.”
There are numerous reasons why product might be rejected – overweight losses, size and shape variances, quality and colour inconsistencies – but, according to Akavia, they are “invisible to the human eye”. He continues: “But the cause is there, somewhere, interwoven within their complex production processes.”
Through its capacity to process thousands upon thousands of data points, taking into account all the interrelationships between them, AI gives companies the ability to reveal hidden root-causes of waste and inefficiency. “This is the awesome potential of industrial AI,” Akavia argues. “It opens up a whole realm of possibilities that simply weren’t possible before.”
Specifically, Seebo’s process-based AI system combines “powerful machine learning algorithms with embedded process expertise”, the company states, enabling “continuous, multivariate analysis of all the data, while fully understanding the unique complexities of each production process”.
Seebo, however, declines to give specific details about the changes in production processes that its technology has brought about at major food companies, for reasons of customer confidentiality.
It includes some testimonials on its website from companies, which offer perspectives on how the food industry is engaging with the tech sector and participating in the Fourth Industrial Revolution, but these are also short on specific detail.
This is perhaps not surprising given the commercial sensitivity of data analytics. Shawn Carver, CEO and co-founder of Canadian tech firm Fiddlehead Technology, believes this also helps explain why AI in manufacturing has been less discussed.
“Because production innovation can lead to significant competitive advantage, it is less likely that companies will discuss these types of initiatives publicly in great detail,” Carver tells just-food.
Both Nestlé and General Mills chose not to engage with this article. However, Mondelez was prepared to offer some further detail. Eugenio Alvarez, engineering associate director at the US-based snacks giant, endorses the application of AI to reduce waste in plants.
“In this case, with the Seebo Process Quality solution, we just achieved 3% overweight reduction,” Alvarez says, adding that equates to savings of $750,000 a year on the single pilot product line for Belvita at its plant in Chicago.
“We have also targeted other waste reduction specifically in broken cookies, size/shape variability, and quality improvement,” Alvarez adds. “We are in the process to collect those benefits as we continue to drive actions taking insights from the AI solution.”
Mondelez also says that AI is supporting predictive maintenance, which prevents machine breakdowns that can often result in waste.
Carver, meanwhile, sees the use of image recognition algorithms to analyse data from cameras, near-infrared spectroscopy, x-rays, and lasers in processing plants as one of the greatest opportunities to use AI technology in food processing. AI provides a means to sort raw material for optimal use and conduct non-destructive quality testing, Carver explains.
In March, Fiddlehead sold a minority stake to Canada-based potato-products major McCain Foods, underlining the close links that some of the larger food companies are establishing with the tech sector through acquisition and investment.
Data challenge
Large multinationals are in a better position than smaller food companies to overcome the most serious limitation faced when contemplating the use of AI technology to reduce food waste – namely its reliance on quality data, and lots of it.
However, Mike von Massow, associate professor in the University of Guelph’s Department of Food, Agricultural and Resource Economics, sees the lack of quality data regarding food waste as a challenge even for many of the large multinationals.
“To a degree, we’ve institutionalised waste. We’ve just accepted that it happens and that means we haven’t measured it very well,” von Massow says. “We have the paradigm that this is the process and that [waste] is the natural outcome of the process.”
This view is echoed by Liz Goodwin, senior fellow and director for food loss and waste at The World Resources Institute. Goodwin sees “huge potential” for AI to play a positive role in reducing food waste in processing. But she adds, while larger companies have recognised the financial benefits and “a clear financial case for investment” in AI technology, some companies “still see waste as just part of doing business”.
However, Akavia suggests a lack of historical data should not be seen as a daunting challenge. “Many baked goods manufacturers don’t have strong data infrastructures, or even any data at all. And this is true even for some of the major manufacturers,” Akavia says.
“For that reason, many manufacturing executives are under the impression that AI is simply beyond them, because the task of building a data infrastructure is just too insurmountable. But that simply isn’t true.”
Akavia says that Seebo has worked on a number of production lines where there was no data whatsoever, and “within just a few months” they had not only built the required data infrastructure, but also deployed AI and started “to see results in terms of reduced production losses”.
A normalised mindset regarding food waste is being constantly challenged by the general increasing concerns about climate change and food security. While the increased computational power of AI reveals hidden causes of inefficiency and waste, von Massow believes it could also help to remove ingrained management biases that accept waste as an unavoidable consequence of a given production process.
The lack of historical data or the necessary levels of automation even to begin data collection is only one among several factors possibly limiting the capacity for SMEs in the food sector to capitalise fully on the potential of AI to help reduce waste. Financial resources are clearly a further significant factor.
Akavia says: “Building a data infrastructure does take work, and it also costs money. But, as long as there is a clear business objective, the effort is relatively low and quickly pays for itself.”
This may well be true but SMEs may lack the capacity even for a relatively short-term investment.
Goodwin sounds an optimistic note, however: “I am sure we still do not know the full potential that AI can play. The technology is developing all the time, which is great to see. To have more use globally, the approaches will need to be adapted to different situations.
“I don’t think we will see ultra-modern plants everywhere in the world for some time to come. However, AI has a role elsewhere, not just in ultra-modern plants.”
The substantial investments in AI by major food companies are to be welcomed and, by dint of these companies’ huge volumes, should make a sizeable contribution to reducing food waste. For AI to realise its full impact on the global food waste challenge however, it will have to be applied far more extensively across food businesses of all sizes.
This feature was initially published in the June 2021 issue of Just Food magazine.
GlobalData analysis: Artificial Intelligence (AI) in Consumer Goods – Thematic Research